What Are the Applications of Statistics in Data Analysis
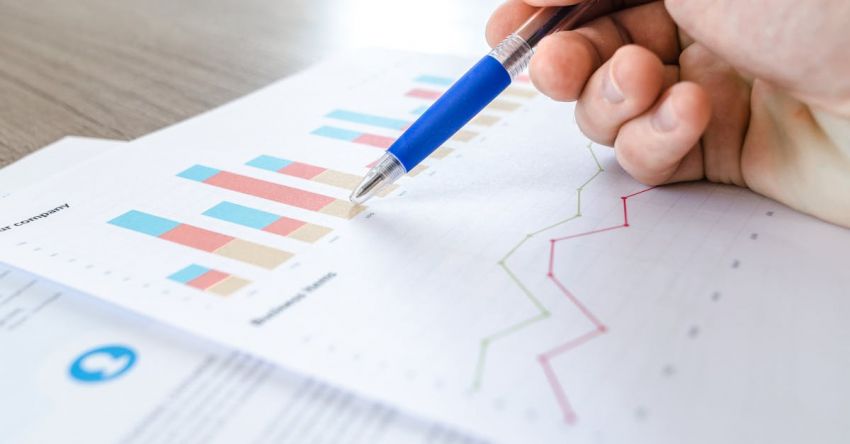
Statistics is a powerful tool that plays a crucial role in data analysis across various fields. By utilizing statistical techniques, analysts can derive valuable insights from large datasets, uncover patterns, and make informed decisions. In today’s data-driven world, the applications of statistics in data analysis are vast and diverse, revolutionizing industries and driving innovation. Let’s delve into the key applications of statistics in data analysis.
Understanding Data Distribution
One of the fundamental applications of statistics in data analysis is understanding data distribution. By analyzing the distribution of data, analysts can gain insights into the central tendency, dispersion, and shape of the dataset. Descriptive statistics such as mean, median, and mode help in summarizing the data, providing a clear picture of the dataset’s characteristics. Histograms, box plots, and scatter plots are commonly used graphical tools to visualize data distribution and identify patterns or outliers.
Hypothesis Testing
Hypothesis testing is a powerful statistical tool used to make inferences about a population based on sample data. In data analysis, hypothesis testing helps analysts determine the significance of relationships or differences between variables. By setting up null and alternative hypotheses and conducting statistical tests, analysts can draw conclusions about the population parameters. Whether it’s testing the effectiveness of a new drug or evaluating the impact of a marketing campaign, hypothesis testing plays a critical role in data analysis.
Predictive Modeling
Predictive modeling is an application of statistics that involves using historical data to make predictions about future events or outcomes. By building statistical models such as regression analysis, time series analysis, or machine learning algorithms, analysts can forecast trends, identify patterns, and make informed decisions. Predictive modeling is widely used in various industries, including finance, healthcare, marketing, and e-commerce, to optimize strategies, reduce risks, and improve decision-making processes.
Cluster Analysis
Cluster analysis is a statistical technique used to group similar objects or data points into clusters based on their characteristics. In data analysis, cluster analysis helps in identifying patterns, segmenting customers, and organizing data into meaningful groups. By using clustering algorithms such as k-means clustering or hierarchical clustering, analysts can uncover hidden patterns and relationships within the dataset. Cluster analysis is valuable for market segmentation, anomaly detection, and pattern recognition in data analysis.
Time Series Analysis
Time series analysis is a statistical method used to analyze and interpret time-dependent data. By studying the patterns, trends, and seasonality in time series data, analysts can make forecasts, detect anomalies, and understand the underlying dynamics of the data. Time series analysis is widely applied in finance, economics, meteorology, and other fields to predict stock prices, sales trends, weather patterns, and more. Techniques such as autocorrelation, moving averages, and exponential smoothing are commonly used in time series analysis.
Statistical Quality Control
Statistical quality control is an application of statistics that focuses on monitoring and improving the quality of processes or products. By using statistical tools such as control charts, process capability analysis, and hypothesis testing, analysts can identify variations, detect defects, and implement corrective actions. Statistical quality control is essential in manufacturing, healthcare, and service industries to ensure consistency, reliability, and efficiency in processes.
In Conclusion
The applications of statistics in data analysis are vast and versatile, empowering analysts to extract meaningful insights, make accurate predictions, and drive informed decisions. From understanding data distribution to predictive modeling, statistics plays a pivotal role in transforming raw data into valuable information. By leveraging statistical techniques and tools, analysts can unlock the potential of data, uncover hidden patterns, and gain a competitive edge in today’s data-driven world.